Ethical and Regulatory Challenges in Generative AI-Driven Healthcare
- Acroplans
- Nov 20, 2024
- 5 min read
Updated: Dec 17, 2024
Generative AI has become a transformative force in healthcare, revolutionizing patient care, diagnostics, and drug discovery. However, with these advancements come significant ethical and regulatory challenges that must be navigated to ensure the responsible and equitable deployment of these technologies.
This blog will delve into the intricate landscape of ethical dilemmas, regulatory requirements, and the implications for the future of AI in healthcare.
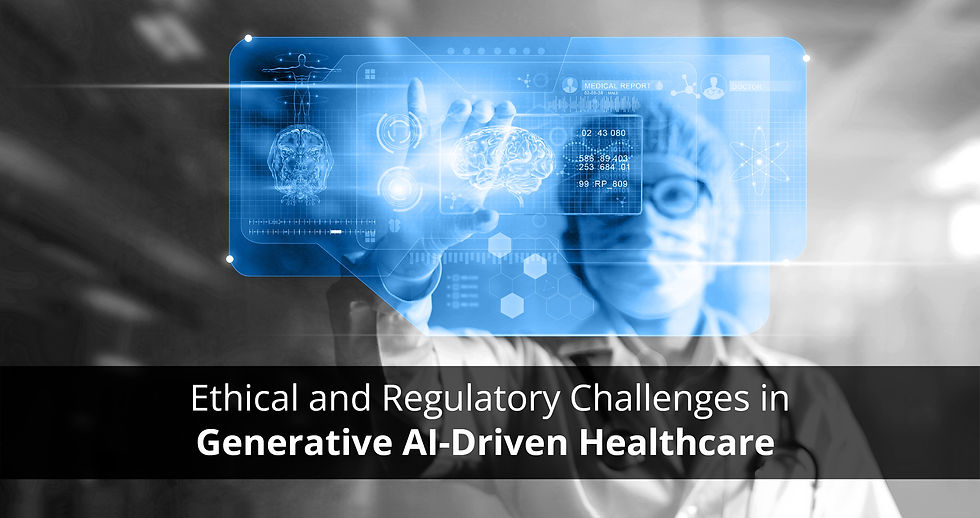
Understanding Generative AI in Healthcare
Generative AI encompasses a range of technologies that leverage algorithms to generate new content based on existing data. In healthcare, this can mean creating realistic patient data for training algorithms, synthesizing new drug compounds, or even generating medical reports. As these applications become more sophisticated, they bring forth challenges that necessitate careful consideration of ethical implications and regulatory frameworks.
The Promise of Generative AI
Before delving into challenges, it’s essential to recognize the promise that generative AI holds for healthcare. From improving patient outcomes through personalized treatment plans to accelerating drug development processes, the potential benefits are vast. For example, generative AI models can analyze vast datasets to identify patterns that humans may overlook, leading to earlier diagnoses of conditions like cancer.
Additionally, by generating synthetic data, healthcare organizations can augment their datasets without compromising patient privacy. This approach not only enhances the robustness of AI models but also alleviates concerns regarding data security. However, while the capabilities of generative AI are remarkable, they must be balanced against ethical considerations.
Ethical Challenges
Patient Privacy and Data Security
One of the foremost ethical challenges in AI-driven healthcare is ensuring patient privacy. As healthcare organizations increasingly rely on patient data to train AI models, the risk of breaches and unauthorized access to sensitive information rises. Generative AI can create realistic patient data for training purposes, but the line between synthetic and real data can blur, leading to potential misuse.
To mitigate these risks, organizations must prioritize data anonymization and implement robust security protocols. Additionally, ethical frameworks should guide the development and deployment of generative AI tools, emphasizing transparency and accountability.
Informed Consent
Informed consent is a cornerstone of ethical medical practice. With generative AI, the question arises: how can patients provide informed consent when the complexities of AI systems may not be fully understood? Healthcare providers must ensure that patients are not only aware of how their data will be used but also understand the implications of AI-driven decisions on their health.
To address this, educational initiatives are crucial. Patients should be provided with clear, accessible information about AI technologies, their benefits, and potential risks. This will empower patients to make informed choices about their participation in AI-driven healthcare systems.
Bias and Fairness
Bias in AI algorithms is another critical ethical issue. If the data used to train AI models is skewed or unrepresentative, the resulting algorithms can perpetuate existing disparities in healthcare. For instance, if an AI model is primarily trained on data from one demographic group, it may not perform well for others, leading to unequal treatment outcomes.
To combat this, it is essential to adopt diverse datasets that accurately reflect the populations being served. Additionally, continuous monitoring and auditing of AI systems should be conducted to identify and rectify biases that may emerge over time.
Accountability and Liability
As AI systems take on more decision-making responsibilities in healthcare, questions of accountability and liability come to the forefront. If an AI system makes a decision that negatively impacts a patient’s health, who is responsible? Is it the healthcare provider, the AI developer, or the organization using the AI?
Establishing clear guidelines for accountability is essential. Stakeholders must engage in discussions to define roles and responsibilities concerning AI-driven decisions, ensuring that patients are protected and that ethical standards are upheld.
Regulatory Challenges
Lack of Comprehensive Regulations
The rapid evolution of generative AI technologies has outpaced the development of regulatory frameworks. Many healthcare organizations operate in a landscape where regulations are ambiguous or nonexistent, leading to inconsistencies in the application of AI technologies.
To address this, regulatory bodies must collaborate with healthcare professionals, technologists, and ethicists to create comprehensive regulations that govern the use of AI in healthcare. These regulations should encompass safety standards, data protection measures, and protocols for clinical validation of AI systems.
Clinical Validation and Approval Processes
The integration of generative AI into clinical practice raises questions about the validation and approval processes for AI-driven tools. Unlike traditional medical devices, AI systems often learn and adapt over time, making it challenging to apply standard approval procedures.
Regulatory agencies need to develop new frameworks for evaluating AI systems, focusing on real-world performance and safety. This may involve post-market surveillance and continuous monitoring to ensure that AI tools remain effective and safe as they are used in clinical settings.
International Variability in Regulations
The global nature of healthcare means that regulations for AI can vary significantly across countries. This variability poses challenges for multinational healthcare organizations that may need to navigate different regulatory landscapes when implementing AI solutions.
To mitigate these challenges, international collaboration is essential. Regulatory bodies should work towards harmonizing standards and regulations for AI in healthcare, ensuring that innovations can be deployed safely and effectively across borders.
Case Studies: Navigating Ethical and Regulatory Challenges
IBM Watson Health
IBM Watson Health has been a pioneer in using AI to assist in medical diagnoses and treatment plans. However, its deployment has not been without controversy. In 2019, reports emerged that Watson was providing unsafe treatment recommendations due to biased training data. This case highlights the ethical implications of relying on AI systems without robust validation and accountability measures.
AI in Radiology
In radiology, AI tools are increasingly used to assist in interpreting medical images. While these tools can enhance diagnostic accuracy, they also raise concerns about accountability. In cases where an AI system fails to detect a malignancy, determining liability becomes complex. The need for clear guidelines and collaborative frameworks between radiologists and AI developers is critical to addressing these challenges.
Generating Synthetic Patient Data
Several organizations have explored the use of synthetic patient data generated by AI to train algorithms while preserving privacy. While this approach holds promise, ethical concerns about the potential misuse of synthetic data remain. Regulatory frameworks must be established to ensure that synthetic data is used responsibly and does not inadvertently compromise patient safety.
Future Directions: Balancing Innovation and Ethics
As generative AI continues to evolve, finding a balance between innovation and ethical considerations is paramount. Healthcare stakeholders must engage in ongoing dialogues to address the challenges posed by AI technologies.
Multidisciplinary Approaches
The complexities of generative AI in healthcare necessitate multidisciplinary approaches that bring together experts from various fields. Ethicists, technologists, healthcare professionals, and regulators should collaborate to develop ethical frameworks and guidelines that promote responsible AI deployment.
Public Engagement and Education
Engaging the public in discussions about AI in healthcare is crucial for fostering trust and understanding. Educational initiatives should aim to inform patients about the benefits and risks associated with AI technologies, empowering them to actively participate in their healthcare decisions.
Continuous Monitoring and Adaptation
The landscape of AI in healthcare is dynamic, requiring continuous monitoring and adaptation of regulatory frameworks. As new challenges emerge, regulatory bodies must be agile and responsive, ensuring that regulations evolve in tandem with technological advancements.
Conclusion
The integration of generative AI in healthcare presents both exciting opportunities and significant ethical and regulatory challenges. By addressing these challenges proactively, stakeholders can harness the transformative potential of AI while safeguarding patient rights and promoting equitable healthcare outcomes. As we move forward, it is essential to prioritize ethics and responsibility in AI development, ensuring that innovation serves the greater good.
Ready to unlock the potential of your business? Contact Acroplans today to learn more about Ethical and Regulatory Challenges in Generative AI-Driven Healthcare and how it can benefit your organization.
Interested?
Schedule a free consultation, our experts are ready to help you reduce cost and risk while innovating with agility.
Comments